38 noisy labels deep learning
Learning from Noisy Labels with Deep Neural Networks: A Survey Abstract: Deep learning has achieved remarkable success in numerous domains with help from large amounts of big data. However, the quality of data labels is a concern because of the lack of high-quality labels in many real-world scenarios. As noisy labels severely degrade the generalization performance of deep neural networks, learning from noisy labels (robust training) is becoming an ... Feature-Induced Label Distribution for Learning with Noisy Labels • Label distributions are more robust than hard labels. • Regularization are beneficial to avoid overfitting. Abstract It is challenging to train deep neural networks robustly with noisy labels, since the deep neural networks can totally over-fit on these noisy labels.
Learning with noisy labels | Papers With Code Deep learning with noisy labels is practically challenging, as the capacity of deep models is so high that they can totally memorize these noisy labels sooner or later during training. 5 Paper Code Generalized Cross Entropy Loss for Training Deep Neural Networks with Noisy Labels AlanChou/Truncated-Loss • • NeurIPS 2018
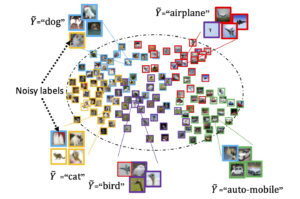
Noisy labels deep learning
Learning from Noisy Labels with Deep Neural Networks: A Survey A two-stage learning method based on noise cleaning to identify and remediate the noisy samples, which improves AUC and recall of baselines by up to 8.9% and 23.4%, respectively and shows that learning from noisy labels can be effective for data-driven software and security analytics. Highly Influenced PDF Learning from Noisy Labels with Deep Neural Networks: A Survey Deep learning has achieved remarkable success in numerous domains with help from large amounts of big data. However, the quality of data labels is a concern because of the lack of high-quality labels in many real-world scenarios. As noisy labels severely degrade the generalization performance of deep neural networks, learning from noisy labels (robust training) is becoming an important task in ... Data fusing and joint training for learning with noisy labels It is well known that deep learning depends on a large amount of clean data. Because of high annotation cost, various methods have been devoted to annotating the data automatically. However, a larger number of the noisy labels are generated in the datasets, which is a challenging problem. In this paper, we propose a new method for selecting training data accurately. Specifically, our approach ...
Noisy labels deep learning. machine learning - Classification with noisy labels ... - Cross Validated Let p t be a vector of class probabilities produced by the neural network and ℓ ( y t, p t) be the cross-entropy loss for label y t. To explicitly take into account the assumption that 30% of the labels are noise (assumed to be uniformly random), we could change our model to produce. p ~ t = 0.3 / N + 0.7 p t. instead and optimize. (PDF) Deep learning with noisy labels: Exploring techniques and ... Training deep learning models with datasets containing noisy labels leads to poor generalization capabilities. Some studies use different deep learning related techniques to improve generalization... PDF O2U-Net: A Simple Noisy Label Detection Approach for Deep Neural Networks •Human Annotations: The combination of noisy label detection and active learning [16] can further benefit supervised learning. In industry, a raw dataset is typi-cally allowed to be verified and annotated for multiple rounds to guarantee its cleanness. Active learning can be conducted after noisy label detection to further re-duce human ... GitHub - songhwanjun/Awesome-Noisy-Labels: A Survey Learning from Noisy Labels with Deep Neural Networks: A Survey This is a repository to help all readers who are interested in handling noisy labels. If your papers are missing or you have other requests, please contact to ghkswns91@gmail.com. We will update this repository and paper on a regular basis to maintain up-to-date.
gorkemalgan/deep_learning_with_noisy_labels_literature This repo consists of collection of papers and repos on the topic of deep learning by noisy labels. All methods listed below are briefly explained in the paper Image Classification with Deep Learning in the Presence of Noisy Labels: A Survey. More information about the topic can also be found on the survey. Deep learning with noisy labels: Exploring techniques and remedies in ... Deep learning with noisy labels: Exploring techniques and remedies in medical image analysis Abstract Supervised training of deep learning models requires large labeled datasets. There is a growing interest in obtaining such datasets for medical image analysis applications. However, the impact of label noise has not received sufficient attention. Deep learning with noisy labels: Exploring techniques and remedies in ... Most of the methods that have been proposed to handle noisy labels in classical machine learning fall into one of the following three categories ( Frénay and Verleysen, 2013 ): 1. Methods that focus on model selection or design. Fundamentally, these methods aim at selecting or devising models that are more robust to label noise. How to Improve Deep Learning Model Robustness by Adding Noise 4. # import noise layer. from keras.layers import GaussianNoise. # define noise layer. layer = GaussianNoise(0.1) The output of the layer will have the same shape as the input, with the only modification being the addition of noise to the values.
PDF Deep Self-Learning From Noisy Labels - CVF Open Access In the following sections, we introduce the iterative self- learning framework in details, where a deep network learns from the original noisy dataset, and then it is trained to cor- rect the noisy labels of images. The corrected labels will supervise the training process iteratively. 3.1. Iterative SelfツュLearning Pipeline. How to handle noisy labels for robust learning from uncertainty Most deep neural networks (DNNs) are trained with large amounts of noisy labels when they are applied. As DNNs have the high capacity to fit any noisy labels, it is known to be difficult to train DNNs robustly with noisy labels. These noisy labels cause the performance degradation of DNNs due to the memorization effect by over-fitting. Impact of Noisy Labels in Learning Techniques: A Survey The method of elimination of noisy labels in deep learning approach is further classified into a robust loss function and modeling latent variable. The statistical-based methods have been discussed in the non-deep learning approach in which mostly algorithms were based on majority voting mechanism, bagging and boosting method, noise rate ... Using Noisy Labels to Train Deep Learning Models on Satellite Imagery Using Noisy Labels to Train Deep Learning Models on Satellite Imagery By Lewis Fishgold on August 5th, 2019 Deep learning models perform best when trained on a large number of correctly labeled examples. The usual approach to generating training data is to pay a team of professional labelers.
Learning from Noisy Labels with Deep Neural Networks: A Survey As noisy labels severely degrade the generalization performance of deep neural networks, learning from noisy labels (robust training) is becoming an important task in modern deep learning applications. In this survey, we first describe the problem of learning with label noise from a supervised learning perspective.
PENCIL: Deep Learning with Noisy Labels - deepai.org Deep learning has achieved excellent performance in various computer vision tasks, but requires a lot of training examples with clean labels. It is easy to collect a dataset with noisy labels, but such noise makes networks overfit seriously and accuracies drop dramatically.
Understanding Deep Learning on Controlled Noisy Labels In "Beyond Synthetic Noise: Deep Learning on Controlled Noisy Labels", published at ICML 2020, we make three contributions towards better understanding deep learning on non-synthetic noisy labels. First, we establish the first controlled dataset and benchmark of realistic, real-world label noise sourced from the web (i.e., web label noise ...
Learning From Noisy Labels With Deep Neural Networks: A Survey | IEEE ... Abstract: Deep learning has achieved remarkable success in numerous domains with help from large amounts of big data. However, the quality of data labels is a concern because of the lack of high-quality labels in many real-world scenarios. As noisy labels severely degrade the generalization performance of deep neural networks, learning from noisy labels (robust training) is becoming an ...
Learning from Noisy Labels for Deep Learning - IEEE 24th International ... This special session is dedicated to the latest development, research findings, and trends on learning from noisy labels for deep learning, including but not limited to: Label noise in deep learning, theoretical analysis, and application Webly supervised visual classification, detection, segmentation, and feature learning
Noisy Labels in Remote Sensing Learning from Noisy Labels in Remote Sensing. Deep learning (DL) based methods have recently seen a rise in popularity in the context of remote sensing (RS) image classification. Most DL models require huge amounts of annotated images during training to optimize all parameters and reach a high-performance during evaluation.
Learning From Noisy Labels With Deep Neural Networks: A Survey Deep learning has achieved remarkable success in numerous domains with help from large amounts of big data. However, the quality of data labels is a concern because of the lack of high-quality labels in many real-world scenarios. As noisy labels severely degrade the generalization performance of dee …
Data Noise and Label Noise in Machine Learning | by Till Richter ... Aleatoric, epistemic and label noise can detect certain types of data and label noise [11, 12]. Reflecting the certainty of a prediction is an important asset for autonomous systems, particularly in noisy real-world scenarios. Confidence is also utilized frequently, though it requires well-calibrated models.
Deep Learning Classification With Noisy Labels | DeepAI 3) Another neural network is learned to detect samples with noisy labels. 4) Deep features are extracted for each sample from the classifier. Some prototypes, representing each class, are learnt or extracted. The samples with features too dissimilar to the prototypes are considered noisy. 2.4 Strategies with noisy labels
Data fusing and joint training for learning with noisy labels It is well known that deep learning depends on a large amount of clean data. Because of high annotation cost, various methods have been devoted to annotating the data automatically. However, a larger number of the noisy labels are generated in the datasets, which is a challenging problem. In this paper, we propose a new method for selecting training data accurately. Specifically, our approach ...

From Research to Production with Deep Semi-Supervised Learning | by Varun Nair | Towards Data ...
Learning from Noisy Labels with Deep Neural Networks: A Survey Deep learning has achieved remarkable success in numerous domains with help from large amounts of big data. However, the quality of data labels is a concern because of the lack of high-quality labels in many real-world scenarios. As noisy labels severely degrade the generalization performance of deep neural networks, learning from noisy labels (robust training) is becoming an important task in ...
Learning from Noisy Labels with Deep Neural Networks: A Survey A two-stage learning method based on noise cleaning to identify and remediate the noisy samples, which improves AUC and recall of baselines by up to 8.9% and 23.4%, respectively and shows that learning from noisy labels can be effective for data-driven software and security analytics. Highly Influenced PDF
Post a Comment for "38 noisy labels deep learning"